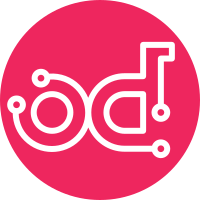
It came to my attention when I was deciding whether or not to use the jenkins_jobs.parallel.parallelize decorator to parallelize things in jenkins_jobs.parser that because it is using the Python threading library nothing is actually parallelized, only concurrentized (at least for CPython). I actually think concurrency is fine for the original use case since that (ie, updating Jenkins jobs) is primarily I/O bound on network connections to a Jenkins instance. However, the "parallel" name really is misleading and could actually be harmful for users of this API who may mistakenly have the impression that it can be used to speed up CPU-bound tasks. Also removes seemingly unnecessary usages of this decorator. ie, jenkins_jobs.builder.Jenkins.changed that is never actually calle with a list of arguments. Change-Id: I996f9dea440e2d6b67ea70870d22942d6eef3ec7
152 lines
5.1 KiB
Python
152 lines
5.1 KiB
Python
#!/usr/bin/env python
|
|
# Copyright (C) 2015 OpenStack, LLC.
|
|
#
|
|
# Licensed under the Apache License, Version 2.0 (the "License");
|
|
# you may not use this file except in compliance with the License.
|
|
# You may obtain a copy of the License at
|
|
#
|
|
# http://www.apache.org/licenses/LICENSE-2.0
|
|
#
|
|
# Unless required by applicable law or agreed to in writing, software
|
|
# distributed under the License is distributed on an "AS IS" BASIS, WITHOUT
|
|
# WARRANTIES OR CONDITIONS OF ANY KIND, either express or implied. See the
|
|
# License for the specific language governing permissions and limitations
|
|
# under the License.
|
|
|
|
# Concurrent execution helper functions and classes
|
|
|
|
from functools import wraps
|
|
import logging
|
|
from multiprocessing import cpu_count
|
|
import threading
|
|
import traceback
|
|
|
|
try:
|
|
import Queue as queue
|
|
except ImportError:
|
|
import queue
|
|
|
|
logger = logging.getLogger(__name__)
|
|
|
|
|
|
class TaskFunc(dict):
|
|
"""
|
|
Simple class to wrap around the information needed to run a function.
|
|
"""
|
|
def __init__(self, n_ord, func, args=None, kwargs=None):
|
|
self['func'] = func
|
|
self['args'] = args or []
|
|
self['kwargs'] = kwargs or {}
|
|
self['ord'] = n_ord
|
|
|
|
|
|
class Worker(threading.Thread):
|
|
"""
|
|
Class that actually does the work, gets a TaskFunc through the queue,
|
|
runs its function with the passed parameters and returns the result
|
|
If the string 'done' is passed instead of a TaskFunc instance, the thread
|
|
will end.
|
|
"""
|
|
def __init__(self, in_queue, out_queue):
|
|
threading.Thread.__init__(self)
|
|
self.in_queue = in_queue
|
|
self.out_queue = out_queue
|
|
|
|
def run(self):
|
|
while True:
|
|
task = self.in_queue.get()
|
|
if task == 'done':
|
|
return
|
|
try:
|
|
res = task['func'](*task['args'],
|
|
**task['kwargs'])
|
|
except Exception as exc:
|
|
res = exc
|
|
traceback.print_exc()
|
|
self.out_queue.put((task['ord'], res))
|
|
|
|
|
|
def concurrent(func):
|
|
@wraps(func)
|
|
def concurrentized(*args, **kwargs):
|
|
"""
|
|
This function will spawn workers and run the decorated function
|
|
concurrently on the workers. It will not ensure the thread safety of
|
|
the decorated function (the decorated function should be thread safe by
|
|
itself). It accepts two special parameters:
|
|
|
|
:arg list concurrentize: list of the arguments to pass to each of the
|
|
runs, the results of each run will be returned in the same order.
|
|
:arg int n_workers: number of workers to use, by default and if '0'
|
|
passed will autodetect the number of cores and use that, if '1'
|
|
passed, it will not use any workers and just run as if were not
|
|
concurrentized everything.
|
|
|
|
Example:
|
|
|
|
> @concurrent
|
|
> def sample(param1, param2, param3):
|
|
> return param1 + param2 + param3
|
|
>
|
|
> sample('param1', param2='val2',
|
|
> concurrent=[
|
|
> {'param3': 'val3'},
|
|
> {'param3': 'val4'},
|
|
> {'param3': 'val5'},
|
|
> ])
|
|
>
|
|
['param1val2val3', 'param1val2val4', 'param1val2val5']
|
|
|
|
This will run the function `concurrentized_function` 3 times, in
|
|
concurrent (depending on the number of detected cores) and return an
|
|
array with the results of the executions in the same order the
|
|
parameters were passed.
|
|
"""
|
|
n_workers = kwargs.pop('n_workers', 0)
|
|
p_kwargs = kwargs.pop('concurrent', [])
|
|
# if only one parameter is passed inside the concurrent dict, run the
|
|
# original function as is, no need for pools
|
|
if len(p_kwargs) == 1:
|
|
kwargs.update(p_kwargs[0])
|
|
if len(p_kwargs) in (1, 0):
|
|
return func(*args, **kwargs)
|
|
|
|
# prepare the workers
|
|
# If no number of workers passed or passed 0
|
|
if not n_workers:
|
|
n_workers = cpu_count()
|
|
logging.debug("Running concurrent %d workers", n_workers)
|
|
worker_pool = []
|
|
in_queue = queue.Queue()
|
|
out_queue = queue.Queue()
|
|
for n_worker in range(n_workers):
|
|
new_worker = Worker(in_queue, out_queue)
|
|
new_worker.setDaemon(True)
|
|
logging.debug("Spawning worker %d", n_worker)
|
|
new_worker.start()
|
|
worker_pool.append(new_worker)
|
|
|
|
# Feed the workers
|
|
n_ord = 0
|
|
for f_kwargs in p_kwargs:
|
|
f_kwargs.update(kwargs)
|
|
in_queue.put(TaskFunc(n_ord, func, args, f_kwargs))
|
|
n_ord += 1
|
|
for _ in range(n_workers):
|
|
in_queue.put('done')
|
|
|
|
# Wait for the results
|
|
logging.debug("Waiting for workers to finish processing")
|
|
results = []
|
|
for _ in p_kwargs:
|
|
new_res = out_queue.get()
|
|
results.append(new_res)
|
|
# cleanup
|
|
for worker in worker_pool:
|
|
worker.join()
|
|
# Reorder the results
|
|
results = [r[1] for r in sorted(results)]
|
|
logging.debug("Concurrent task finished")
|
|
return results
|
|
return concurrentized
|