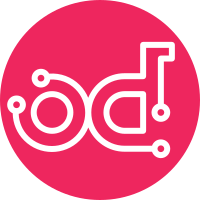
* Only update_jobs uses the parallel features right now * --workers N - If set to 0, it will use parallel execution and use the number of cores in the machine as thread count - Any other value enables the parallel extensions and sets the number of concurrent threads to that value - Will use 1 by default Add some tests to make sure the parallel execution helpers work as expected. Change-Id: Ib0abd34ea7525f75fff4ff480287a6e589deba90 Signed-off-by: David Caro <dcaroest@redhat.com>
152 lines
5.1 KiB
Python
152 lines
5.1 KiB
Python
#!/usr/bin/env python
|
|
# Copyright (C) 2015 OpenStack, LLC.
|
|
#
|
|
# Licensed under the Apache License, Version 2.0 (the "License");
|
|
# you may not use this file except in compliance with the License.
|
|
# You may obtain a copy of the License at
|
|
#
|
|
# http://www.apache.org/licenses/LICENSE-2.0
|
|
#
|
|
# Unless required by applicable law or agreed to in writing, software
|
|
# distributed under the License is distributed on an "AS IS" BASIS, WITHOUT
|
|
# WARRANTIES OR CONDITIONS OF ANY KIND, either express or implied. See the
|
|
# License for the specific language governing permissions and limitations
|
|
# under the License.
|
|
|
|
# Parallel execution helper functions and classes
|
|
|
|
from functools import wraps
|
|
import logging
|
|
from multiprocessing import cpu_count
|
|
import threading
|
|
import traceback
|
|
|
|
try:
|
|
import Queue as queue
|
|
except ImportError:
|
|
import queue
|
|
|
|
logger = logging.getLogger(__name__)
|
|
|
|
|
|
class TaskFunc(dict):
|
|
"""
|
|
Simple class to wrap around the information needed to run a function.
|
|
"""
|
|
def __init__(self, n_ord, func, args=None, kwargs=None):
|
|
self['func'] = func
|
|
self['args'] = args or []
|
|
self['kwargs'] = kwargs or {}
|
|
self['ord'] = n_ord
|
|
|
|
|
|
class Worker(threading.Thread):
|
|
"""
|
|
Class that actually does the work, gets a TaskFunc through the queue,
|
|
runs its function with the passed parameters and returns the result
|
|
If the string 'done' is passed instead of a TaskFunc instance, the thread
|
|
will end.
|
|
"""
|
|
def __init__(self, in_queue, out_queue):
|
|
threading.Thread.__init__(self)
|
|
self.in_queue = in_queue
|
|
self.out_queue = out_queue
|
|
|
|
def run(self):
|
|
while True:
|
|
task = self.in_queue.get()
|
|
if task == 'done':
|
|
return
|
|
try:
|
|
res = task['func'](*task['args'],
|
|
**task['kwargs'])
|
|
except Exception as exc:
|
|
res = exc
|
|
traceback.print_exc()
|
|
self.out_queue.put((task['ord'], res))
|
|
|
|
|
|
def parallelize(func):
|
|
@wraps(func)
|
|
def parallelized(*args, **kwargs):
|
|
"""
|
|
This function will spawn workers and run the decorated function in
|
|
parallel on the workers. It will not ensure the thread safety of the
|
|
decorated function (the decorated function should be thread safe by
|
|
itself). It accepts two special parameters:
|
|
|
|
:arg list parallelize: list of the arguments to pass to each of the
|
|
runs, the results of each run will be returned in the same order.
|
|
:arg int n_workers: number of workers to use, by default and if '0'
|
|
passed will autodetect the number of cores and use that, if '1'
|
|
passed, it will not use any workers and just run as if were not
|
|
parallelized everything.
|
|
|
|
Example:
|
|
|
|
> @parallelize
|
|
> def sample(param1, param2, param3):
|
|
> return param1 + param2 + param3
|
|
>
|
|
> sample('param1', param2='val2',
|
|
> parallelize=[
|
|
> {'param3': 'val3'},
|
|
> {'param3': 'val4'},
|
|
> {'param3': 'val5'},
|
|
> ])
|
|
>
|
|
['param1val2val3', 'param1val2val4', 'param1val2val5']
|
|
|
|
This will run the function `parallelized_function` 3 times, in
|
|
parallel (depending on the number of detected cores) and return an
|
|
array with the results of the executions in the same order the
|
|
parameters were passed.
|
|
"""
|
|
n_workers = kwargs.pop('n_workers', 0)
|
|
p_kwargs = kwargs.pop('parallelize', [])
|
|
# if only one parameter is passed inside the parallelize dict, run the
|
|
# original function as is, no need for pools
|
|
if len(p_kwargs) == 1:
|
|
kwargs.update(p_kwargs[0])
|
|
if len(p_kwargs) in (1, 0):
|
|
return func(*args, **kwargs)
|
|
|
|
# prepare the workers
|
|
# If no number of workers passed or passed 0
|
|
if not n_workers:
|
|
n_workers = cpu_count()
|
|
logging.debug("Running parallel %d workers", n_workers)
|
|
worker_pool = []
|
|
in_queue = queue.Queue()
|
|
out_queue = queue.Queue()
|
|
for n_worker in range(n_workers):
|
|
new_worker = Worker(in_queue, out_queue)
|
|
new_worker.setDaemon(True)
|
|
logging.debug("Spawning worker %d", n_worker)
|
|
new_worker.start()
|
|
worker_pool.append(new_worker)
|
|
|
|
# Feed the workers
|
|
n_ord = 0
|
|
for f_kwargs in p_kwargs:
|
|
f_kwargs.update(kwargs)
|
|
in_queue.put(TaskFunc(n_ord, func, args, f_kwargs))
|
|
n_ord += 1
|
|
for _ in range(n_workers):
|
|
in_queue.put('done')
|
|
|
|
# Wait for the results
|
|
logging.debug("Waiting for workers to finish processing")
|
|
results = []
|
|
for _ in p_kwargs:
|
|
new_res = out_queue.get()
|
|
results.append(new_res)
|
|
# cleanup
|
|
for worker in worker_pool:
|
|
worker.join()
|
|
# Reorder the results
|
|
results = [r[1] for r in sorted(results)]
|
|
logging.debug("Parallel task finished")
|
|
return results
|
|
return parallelized
|