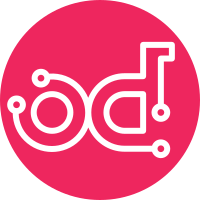
all transformers will now require a grouping key. the pipeline will take grouping keys from all transformers in it's definition. using the set of keys, the values will be pulled from the datapoint, hashed, and sent to a pipeline. if no transformers are applied, samples will be grouped by resource_id and events will be grouped by event_type. Implement blueprint distributed-coordinated-notifications Change-Id: Ief462d19655c238e5951881a58e183084d37ac13
45 lines
1.3 KiB
Python
45 lines
1.3 KiB
Python
#
|
|
# Copyright 2013 Julien Danjou
|
|
#
|
|
# Licensed under the Apache License, Version 2.0 (the "License"); you may
|
|
# not use this file except in compliance with the License. You may obtain
|
|
# a copy of the License at
|
|
#
|
|
# http://www.apache.org/licenses/LICENSE-2.0
|
|
#
|
|
# Unless required by applicable law or agreed to in writing, software
|
|
# distributed under the License is distributed on an "AS IS" BASIS, WITHOUT
|
|
# WARRANTIES OR CONDITIONS OF ANY KIND, either express or implied. See the
|
|
# License for the specific language governing permissions and limitations
|
|
# under the License.
|
|
|
|
from ceilometer import transformer
|
|
|
|
|
|
class TransformerAccumulator(transformer.TransformerBase):
|
|
"""Transformer that accumulates samples until a threshold.
|
|
|
|
And then flushes them out into the wild.
|
|
"""
|
|
|
|
grouping_keys = ['resource_id']
|
|
|
|
def __init__(self, size=1, **kwargs):
|
|
if size >= 1:
|
|
self.samples = []
|
|
self.size = size
|
|
super(TransformerAccumulator, self).__init__(**kwargs)
|
|
|
|
def handle_sample(self, context, sample):
|
|
if self.size >= 1:
|
|
self.samples.append(sample)
|
|
else:
|
|
return sample
|
|
|
|
def flush(self, context):
|
|
if len(self.samples) >= self.size:
|
|
x = self.samples
|
|
self.samples = []
|
|
return x
|
|
return []
|