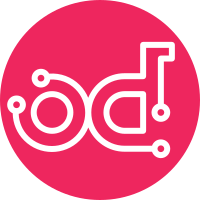
Scan the ceilometer specs repository. Filter the result and fix the mistakes. Change-Id: Idfbc41c3b681aa57cd5153dffc2dae600a58efb9
171 lines
4.8 KiB
ReStructuredText
171 lines
4.8 KiB
ReStructuredText
..
|
|
This work is licensed under a Creative Commons Attribution 3.0 Unported
|
|
License.
|
|
|
|
http://creativecommons.org/licenses/by/3.0/legalcode
|
|
|
|
================================================================
|
|
Using aggregation pipeline instead of map-reduce job in MongoDB
|
|
================================================================
|
|
|
|
https://blueprints.launchpad.net/ceilometer/+spec/mongodb-aggregation-pipeline
|
|
|
|
Problem description
|
|
===================
|
|
|
|
Currently, when we make a GET "/v2/meter/<meter_type/statistics" with MongoDB
|
|
backend it starts a native map-reduce job in MongoDB instance. Tests and deep
|
|
researching show that the job have a lack of performance in work with huge
|
|
amount of samples (several millions and above). For example, job processes
|
|
~10000 samples per second on my test environment (16 GB RAM, 8 CPU, 1 TB disk,
|
|
15000000 samples). So job for 15M samples works ~1500 seconds. It's longer
|
|
than default api timeout, 1 minute.
|
|
|
|
Of course, with Gnocchi dispatcher we haven't issue with statistics, but
|
|
users which are going to use only MongoDB backend will have troubles with alarm
|
|
work and making user reports.
|
|
|
|
|
|
Proposed change
|
|
===============
|
|
|
|
Add an implementation of method get_meter_statistics via MongoDB
|
|
aggregation pipeline framework.
|
|
|
|
From MongoDB docs:
|
|
"This framework modeled on the concept of data processing pipelines. Documents
|
|
enter a multi-stage pipeline that transforms the documents into an aggregated
|
|
result. The most basic pipeline stages provide filters that operate like
|
|
queries and document transformations that modify the form of the output
|
|
document. Other pipeline operations provide tools for grouping and sorting
|
|
documents by specific field or fields as well as tools for aggregating the
|
|
contents of arrays, including arrays of documents. In addition, pipeline stages
|
|
can use operators for tasks such as calculating the average or concatenating a
|
|
string. The pipeline provides efficient data aggregation using native
|
|
operations within MongoDB, and is the preferred method for data aggregation
|
|
in MongoDB."
|
|
|
|
My researches show that aggregation pipeline is faster than native map-reduce
|
|
job to ~10 times. So processing of 15M samples in the same test environment
|
|
works 128 seconds vs 1500 seconds with map-reduce.
|
|
|
|
Pipeline aggregation framework has a large functionality and
|
|
amount of operators, that allows to provide support of all existence
|
|
"statistics" features.
|
|
|
|
This implementation affects only performance of statistics request in
|
|
Ceilometer MongoDB and doesn't affects API or different backends.
|
|
|
|
Risks:
|
|
|
|
This framework have specified limits. It restricted by 100 MB RAM for stage
|
|
otherwise it needs to write temporary files with intermediate stages results
|
|
to disk. For avoiding failing caused by excessive memory using in MongoDB>=2.6
|
|
we can use the option `allowDiskUse=True` in aggregation command .
|
|
This option allows to write intermediate staging data to temporary files.
|
|
So, primary risks of this approach are a necessity of free space
|
|
on disk and a slow performance of disk writing and reading.
|
|
|
|
Accordingly, researches and MongoDB docs, the "$sort" command creates
|
|
the most amount of intermediate data for follow stages. So, in practice
|
|
this stage prepares data whose size is close to new index size.
|
|
In same time, the indexed fields sorting (like timestamp
|
|
in our `meter` collection) does not need the any additional data in the disk.
|
|
This request uses the existing index for sorting.
|
|
Other commands works with processed and grouped data and use
|
|
additional space only in worst case (huge amount of resources and
|
|
group by resource_id in one request).
|
|
|
|
Despite to writing temporary file into disk the aggregation command
|
|
in this case faster than Map-Reduce up to several times.
|
|
|
|
Also this MongoDB mechanisms have limit in size
|
|
of finally document in 16 MB, same as map-reduce job.
|
|
|
|
|
|
Alternatives
|
|
------------
|
|
|
|
Also we may improve performance of map-reduce job
|
|
|
|
Data model impact
|
|
-----------------
|
|
|
|
None
|
|
|
|
REST API impact
|
|
---------------
|
|
|
|
None
|
|
|
|
Security impact
|
|
---------------
|
|
|
|
None
|
|
|
|
Pipeline impact
|
|
---------------
|
|
|
|
None
|
|
|
|
Other end user impact
|
|
---------------------
|
|
|
|
None
|
|
|
|
Performance/Scalability Impacts
|
|
-------------------------------
|
|
|
|
Improve performance of GET "/v2/<meter_name>/statistics" request.
|
|
|
|
Other deployer impact
|
|
---------------------
|
|
|
|
None
|
|
|
|
Developer impact
|
|
----------------
|
|
|
|
None
|
|
|
|
Implementation
|
|
==============
|
|
|
|
Assignee(s)
|
|
-----------
|
|
|
|
Primary assignee:
|
|
ityaptin
|
|
|
|
Ongoing maintainer:
|
|
idegtiarev
|
|
|
|
Work Items
|
|
----------
|
|
|
|
- implement a get_meter_statistics function with aggregation pipeline framework
|
|
|
|
Future lifecycle
|
|
================
|
|
|
|
None
|
|
|
|
Dependencies
|
|
============
|
|
|
|
None
|
|
|
|
Testing
|
|
=======
|
|
|
|
- current tests are check correct work of "statistics" request
|
|
|
|
Documentation Impact
|
|
====================
|
|
|
|
None
|
|
|
|
References
|
|
==========
|
|
http://docs.mongodb.org/v2.4/core/aggregation-introduction/
|