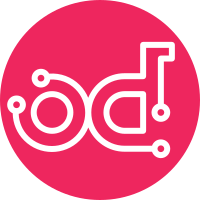
Class docstrings were being pulled in to multiple locations, causing sphinx errors due to the duplicate references. This fixes it by adding the :noindex: option to those references. Also temporarily disabling the PDF build as that is failing with another issue that should be fixed in a follow up. Change-Id: I6de2948ff49e1bb0b6a8b9a3a90f9f2ebbb8b7bb Signed-off-by: Sean McGinnis <sean.mcginnis@gmail.com>
5.2 KiB
Collector
Data format
Internally, CloudKitty's data format is a bit more detailled than what can be found in the architecture documentation.
The internal data format is the following:
{
"bananas": [
{
"vol": {
"unit": "banana",
"qty": 1
},
"rating": {
"price": 1
},
"groupby": {
"xxx_id": "hello",
"yyy_id": "bye",
},
"metadata": {
"flavor": "chocolate",
"eaten_by": "gorilla",
},
}
],
}
However, developers implementing a collector don't need to format the data themselves, as there are helper functions for these matters.
Implementation
Each collector must implement the following class:
cloudkitty.collector.BaseCollector
The retrieve
method of the BaseCollector
class is called by the orchestrator. This method calls the
fetch_all
method of the child class.
To create a collector, you need to implement at least the
fetch_all
method.
Data collection
Collectors must implement a fetch_all
method. This
method is called for each metric type, for each scope, for each collect
period. It has the following prototype:
cloudkitty.collector.BaseCollector
This method is supposed to return a list of
cloudkitty.dataframe.DataPoint
objects.
Example code of a basic collector:
from cloudkitty.collector import BaseCollector
class MyCollector(BaseCollector):
def __init__(self, **kwargs):
super(MyCollector, self).__init__(**kwargs)
def fetch_all(self, metric_name, start, end,
=None, q_filter=None):
project_id= []
data for CONDITION:
# do stuff
data.append(dataframe.DataPoint(
unit,# int, float, decimal.Decimal or str
qty, 0, # price
# dict
groupby, # dict
metadata,
))
return data
project_id
can be misleading, as it is a legacy name. It
contains the ID of the current scope. The attribute corresponding to the
scope is specified in the configuration, under
[collect]/scope_key
. Thus, all queries should filter based
on this attribute. Example:
from oslo_config import cfg
from cloudkitty.collector import BaseCollector
= cfg.CONF
CONF
class MyCollector(BaseCollector):
def __init__(self, **kwargs):
super(MyCollector, self).__init__(**kwargs)
def fetch_all(self, metric_name, start, end,
=None, q_filter=None):
project_id= CONF.collect.scope_key
scope_key = {'start': start, 'stop': stop, scope_key: project_id}
filters
= self.client.query(
data =filters,
filters=self.conf[metric_name]['groupby'])
groupby# Format data etc
return output
Additional configuration
If you need to extend the metric configuration (add parameters to the
extra_args
section of metrics.yml
), you can
overload the check_configuration
method of the base
collector:
cloudkitty.collector.BaseCollector
This method uses voluptuous for data
validation. The base schema for each metric can be found in
cloudkitty.collector.METRIC_BASE_SCHEMA
. This schema is
meant to be extended by other collectors. Example taken from the gnocchi
collector code:
from cloudkitty import collector
= {
GNOCCHI_EXTRA_SCHEMA 'extra_args'): {
Required('resource_type'): All(str, Length(min=1)),
Required(# Due to Gnocchi model, metric are grouped by resource.
# This parameter allows to adapt the key of the resource identifier
'resource_key', default='id'): All(str, Length(min=1)),
Required('aggregation_method', default='max'):
Required('max', 'mean', 'min']),
In([
},
}
class GnocchiCollector(collector.BaseCollector):
= 'gnocchi'
collector_name
@staticmethod
def check_configuration(conf):
= collector.BaseCollector.check_configuration(conf)
conf = Schema(collector.METRIC_BASE_SCHEMA).extend(
metric_schema
GNOCCHI_EXTRA_SCHEMA)
= {}
output for metric_name, metric in conf.items():
= output[metric_name] = metric_schema(metric)
met
if met['extra_args']['resource_key'] not in met['groupby']:
'groupby'].append(met['extra_args']['resource_key'])
met[
return output
If your collector does not need any extra_args
, it is
not required to overload the check_configuration
method.